Via Google Scholar, I noticed the following two citations to the DSSR-enabled innovative schematics of 3D nucleic acid structures with PyMOL paper recently published in Nucleic Acids Research (NAR):
Here are the direct quotations on the DSSR-PyMOL paper from these two citations.
DSSR [38] processes the 3D structure of the RNA molecule and annotates its secondary structure. It is a part of the 3DNA suite [67] designed to work with the structures of nucleic acids. DSSR identifies, classifies and describes base pairs, multiplets and characteristic motifs of the secondary structure; helices, stems, hairpin loops, bulges, internal loops, junctions and others. It can also detect modules and tertiary structure patterns, including pseudoknots and kink-turns. The recent extension, DSSR-PyMOL [68], allows drawing cartoon-block schemes of the 3D structure and responds to the need for simplified visualization of quadruplexes.
DSSR-PyMOL generated block schemes of both quadruplexes (Figure 4A3 and B3).
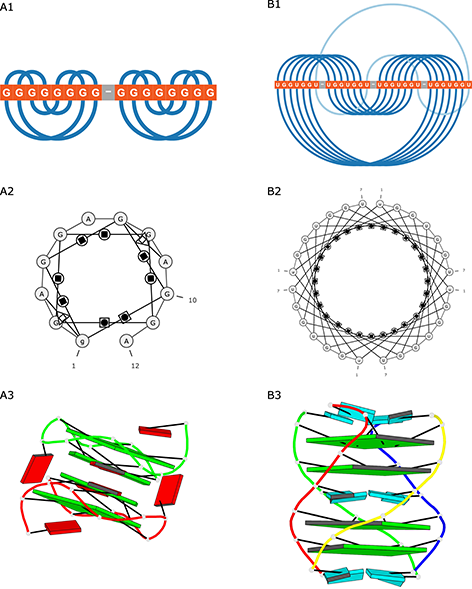
Figure 4: Visualization of (A) 2RQJ and (B) 6GE1 structures generated by (1) ElTetrado, (2) RNApdbee and (3) DSSR-PyMOL.
Next, the structural model of the N-NTD:dsTRS (5’–UCUAAAC–3’) complex was generated from the lowest-energy structure of the N-NTD:dsNS complex, derived from the cluster with the lowest HADDOCK score, by mutating the dsRNA sequence using w3DNA (29). Therefore, both complexes have identical geometries, varying only the dsRNA sequences. Structural conformation of the constructed model for N-NTD:dsTRS complex was displayed using the web application http://skmatic.x3dna.org for easy creation of DSSR (Dissecting the Spatial Structure of RNA)-PyMOL schematics (32).
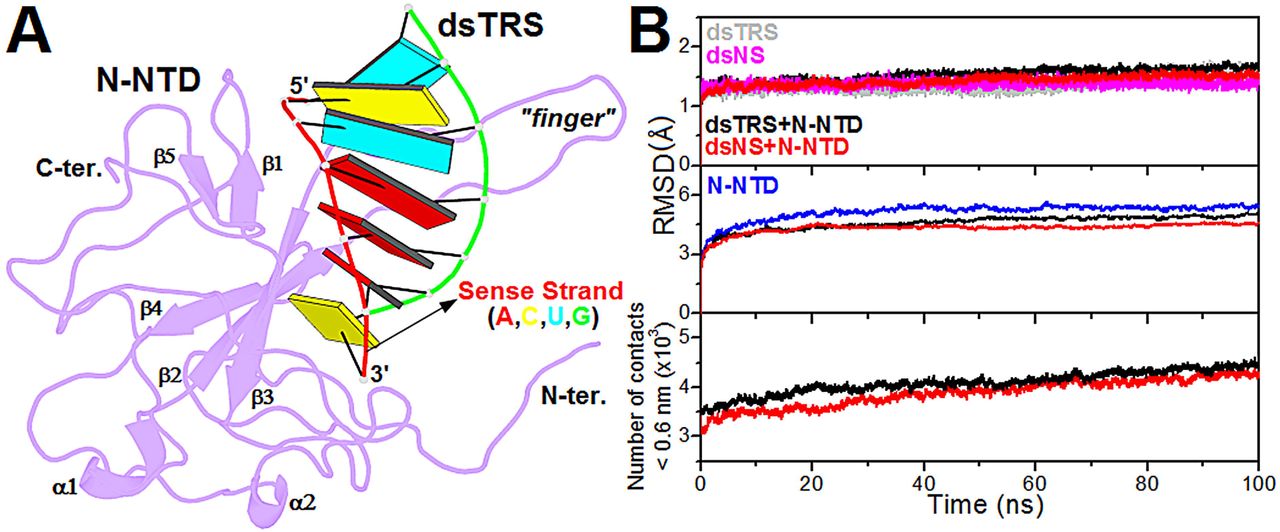
Figure 1: Structural model of the N-NTD:dsRNA complex and its validation from molecular dynamics simulations. (A) Structural model of the N-NTD:dsTRS complex determined by molecular docking calculations and mutation of dsNS nucleotide sequence. N-NTD is presented as purple cartoon and dsTRS is denoted as a ribbon model with base pairing as colored rectangles. The color of the rectangles corresponds to the nitrogenous base of the dsRNA sense strand, namely A: red, C: yellow, U: cyan, and G: green. The large protruding β2-β3 loop is referred to as the finger. (B) …
p(clean)=. 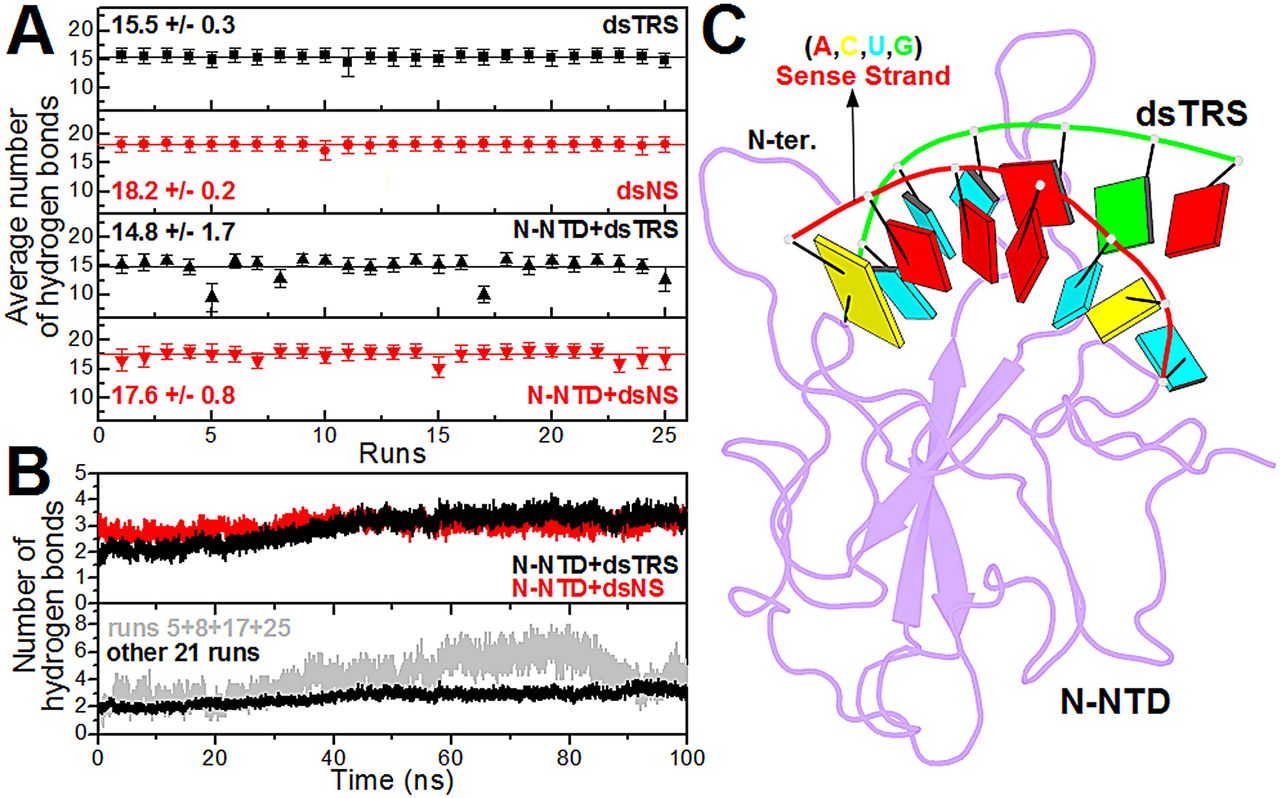
Figure 3: Analysis of the intramolecular (dsRNAs) and intermolecular (N-NTD:dsRNAs) hydrogen bonds. (A) … (B) … (C) Structural model of the N-NTD:dsTRS complex representative of the MD simulation for run 5. The protein is shown in purple cartoon and dsTRS is denoted as a ribbon model with nitrogenous bases and base-pairing as colored squares and rectangles, respectively. The color of the squares corresponds to the type of nitrogenous base, namely A: red, C: yellow, U: cyan, and G: green, while the rectangles refer to the nitrogenous base color of the dsRNA sense strand.
It is really a pleasure to see the DSSR-PyMOL paper being cited quickly after its publication. I am always curious to see how DSSR is cited in literature. Indeed, over the years following citations to DSSR has become an effective way for me to become informed of directly relevant references. Reading these citing articles motivates me to further improve DSSR.
I recently come across the article FMN riboswitch aptamer symmetry facilitates conformational switching through mutually exclusive coaxial stacking configurations by Wilt et al. in the Journal of Structural Biology: X (JSBX). In the caption to Figure S1, “Secondary structure map of the FMN riboswitch”, the authors wrote:
Base-pairing is annotated using Leontis-Westhoff nomenclature (Leontis and Westhof, 2001), derived using 3DNA-DSSR (Lu and Olson, 2003), and the map was generated using VARNA (Darty et al., 2009).
It is a nice surprise to see that 3DNA-DSSR is cited this way. The LW scheme is based on the three edges of each base with potential for H-bonding interactions (Watson-Crick, Hoogsteen, and Sugar), and the two orientations (cis or trans) of the interacting bases with respect to the glycosidic bonds. The combinations of edges and orientations (3 × 2 × 2) “gives rise to 12 basic geometric types with at least two H bonds connecting the bases” (Leontis and Westhof, 2001). This geometry-based approach captures salient features of pairing interactions and strikes a balance between simplicity and expressiveness. The LW scheme is more widely applicable than the Saenger classification, and more intuitive to biologists. As a result, the LW classification has become a standard in RNA structural bioinformatics.
However, the RNA-centric LW classification has inherent limitations. For example, the Sugar edge explicitly includes the 2′-hydroxyl group, rendering it less applicable to DNA structures. Additionally, while the aromatic base can be taken as a rigid body with three fixed edges, the χ (chi) torsion angle characterizes the internal freedom between base and sugar (anti vs. syn). When χ is in the relatively rare (but not uncommon) syn conformation (especially abundant in G-quadruplexes), the Sugar edge, defined with reference to the common anti conformation, seems to no longer exist. The rich variety of RNA pairs extends beyond the 12 basic LW types. There are numerous pairs in RNA with only one H-bond or with bifurcated H-bonds, at boundary locations where the LW classification does not strictly apply. Lemieux and Major (2002) were the first to extend the LW classification. We noted the importance of the out-of-plane ‘backbone edge’ formed by an RNA-specific H-bond between O2′(G) and OP2 (Lu et al., 2010). Finally, the RNA 3D Hub website, hosted by the Leontis-Zirbel team, lists pairing interactions that do not fall into the 12 geometric types. For example, the page for 1msy contains pairing types ncSW, ntSH, and ntHH. Note that the terms nc (in ncSW) and nt (in ntSH/ntHH) do not have the normal meanings in literature; they stands for near cis and near trans respectively.
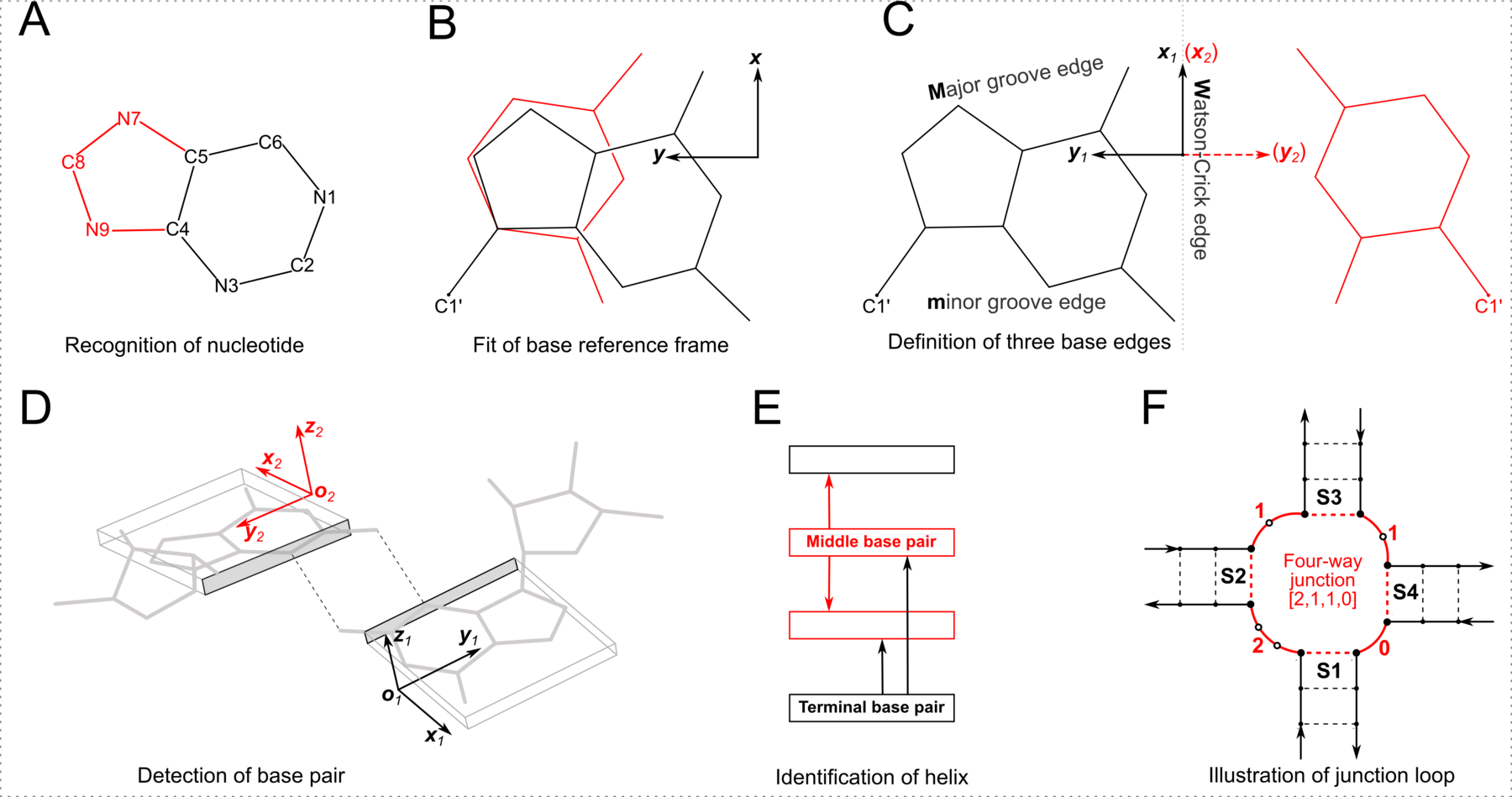
As shown in the figure above, DSSR adopts a base-centric terminology for the three edges. In principle, M (Major groove) in the DSSR classification corresponds to the Hoogsteen/CH-edge (H) in the LW notation, and the DSSR m (minor groove) to the LW Sugar-edge (S) if χ is in the anti conformation. In practice, direct DSSR/LW correspondences M/H and m/S are assumed, regardless of anti/syn base conformation. Moreover, the cis/trans assignment is the same for both notations. Within the DSSR implementation, the LW and DSSR classifications are thus strictly parallel in terms of cis/trans orientation and interacting edges. The DSSR scheme has the extra ± for relative base orientations.
The LW classifications implemented in DSSR may differ from those listed in the RNA 3D Hub website or other resources. These discrepancies normally occur in boundary cases where the assignment of cis/trans and interaction edges can be ambiguous. For ‘authentic’ LW classification results, users should consult the original publication of Leontis and Westhof (2001) and use the RNAView (Yang et al., 2003) or FR3D (Sarver et al., 2008) tools instead of DSSR.
I recently read the paper RIC-seq for global in situ profiling of RNA–RNA spatial interactions published in Nature by the Yuanchao Xue team from the Chinese Academy of Sciences. The abstract is as below:
Highly structured RNA molecules usually interact with each other, and associate with various RNA-binding proteins, to regulate critical biological processes. However, RNA structures and interactions in intact cells remain largely unknown. Here, by coupling proximity ligation mediated by RNA-binding proteins with deep sequencing, we report an RNA in situ conformation sequencing (RIC-seq) technology for the global profiling of intra- and intermolecular RNA–RNA interactions. This technique not only recapitulates known RNA secondary structures and tertiary interactions, but also facilitates the generation of three-dimensional (3D) interaction maps of RNA in human cells. Using these maps, we identify noncoding RNA targets globally, and discern RNA topological domains and trans-interacting hubs. We reveal that the functional connectivity of enhancers and promoters can be assigned using their pairwise-interacting RNAs. Furthermore, we show that CCAT1-5L—a super-enhancer hub RNA—interacts with the RNA-binding protein hnRNPK, as well as RNA derived from the MYC promoter and enhancer, to boost MYC transcription by modulating chromatin looping. Our study demonstrates the power and applicability of RIC-seq in discovering the 3D structures, interactions and regulatory roles of RNA.
The Methods part contains the following section, where DSSR is cited along with several other software tools:
Structural analysis of 28S rRNA. The RIC-seq reads aligned to 45S pre-rRNA (NR_046235.3) were collected and used to construct the interaction matrix shown in Fig. 1h. A Knight–Ruiz normalization al- gorithm, widely used in the normalization of Hi-C contact matrices51, was applied to eliminate sequencing bias along rRNA. For building the physical interaction map of 28S rRNA, the cryo-EM model of human 80S ribosome (RCSB Protein Data Bank (PDB) ID 4V6X) was down- loaded, and the spatial distances between every 5-nt bin in 28S rRNA were calculated using the mean spatial coordinates of carbon atoms in each 5-nt bin. Watson–Crick and non-Watson–Crick base pairs were identified using the DSSR software52. The 3D structure of the ribosome was visualized by the PyMOL system (Educational version, https:// pymol.org/2/). For the missing structures in 28S rRNA, we combined intramolecular RNA–RNA interactions detected by RIC-seq with the RNAstructure algorithm53 to deduce their 2D structures.
There are several other well-known programs for identifying and annotating RNA base pairs, including RNAView, FR3D, and MC-Annotate. One may wonder why DSSR is used here. In addition to asking the authors, interested viewers could simply test for themselves: try the different tools on PDB entry 4V6X and see what happens.
It is worth mentioning that a new DSSR-related paper “DSSR-enabled innovative schematics of 3D nucleic acid structures with PyMOL” has recently been accepted by publication in Nucleic Acids Research. I will shortly write another post on this topic when this paper is officially published online. To see DSSR-PyMOL schematics in action, please visit http://skmatic.x3dna.org. Here is the abstract of the new DSSR-PyMOL article:
Sophisticated analysis and simplified visualization are crucial for understanding complicated structures of biomacromolecules. DSSR (Dissecting the Spatial Structure of RNA) is an integrated computational tool that has streamlined the analysis and annotation of 3D nucleic acid structures. The program creates schematic block representations in diverse styles that can be seamlessly integrated into PyMOL and complement its other popular visualization options. In addition to portraying individual base blocks, DSSR can draw Watson-Crick pairs as long blocks and highlight the minor-groove edges. Notably, DSSR can dramatically simplify the depiction of G-quadruplexes by automatically detecting G-tetrads and treating them as large square blocks. The DSSR-enabled innovative schematics with PyMOL are aesthetically pleasing and highly informative: the base identity, pairing geometry, stacking interactions, double-helical stems, and G-quadruplexes are immediately obvious. These features can be accessed via four interfaces: the command-line interface, the DSSR plugin for PyMOL, the web application, and the web application programming interface. The supplemental PDF serves as a practical guide, with complete and reproducible examples. Thus, even beginners or occasional users can get started quickly, especially via the web application at http://skmatic.x3dna.org.
Recently I read with great interest the article High-Resolution Structure of Cas13b and Biochemical Characterization of RNA Targeting and Cleavage by Slaymaker et al., published in Cell Reports (2019, 26, 3741–3751). This 1.65-Å structure (PDB id: 6dtd) “provides a mechanistic model for Cas13b target RNA recognition and identifies features responsible for target and cleavage specificity.”
I am pleased to see that DSSR is listed in the “KEY RESOURCES TABLE” under the category “Software and Algorithms”, and mentioned in the “Structure Analysis” section:
RNA structure was analyzed using DSSR (Lu et al., 2015). Protein conservation mapping to the structure was done using the Consurf server (Ashkenazy et al., 2016). Protein secondary structure was analyzed using the PDBSUM webserver (de Beer et al., 2014) (Figure S1E). APBS as part of the PyMOL visualization program was used to calculate electrostatics (Jurrus et al., 2018). Structure validation statistics were generated with MolProbity (Chen et al., 2010)
In the main text, the authors cited DSSR for the detection of a base multiplet. Running DSSR on PDB entry 6dtd, I found two base triplets, as shown below:
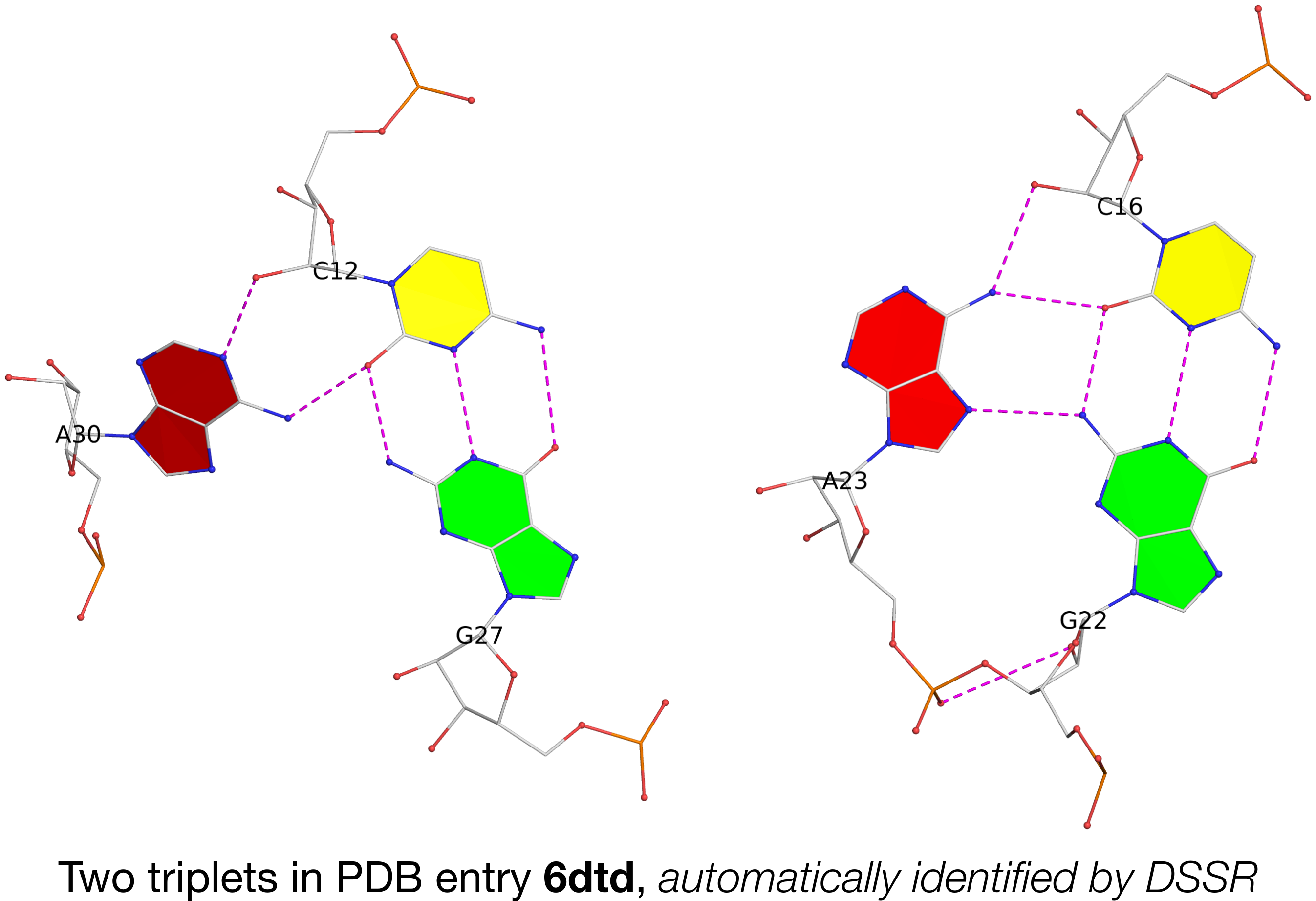
In the figure above, each of the two adenines is interacting with a G–C pair in the minor-groove edge (m) of the pair: A30 (left) is using its Watson-Crick edge (W), whilst A23 (right) is employing its major-groove edge (M). Thus they do not belong to the canonical A-minor motifs (types I or II) where the minor-groove edge of A interacts with the minor-groove edge of a WC pair. In DSSR, they are classified as type=X
, a general category of noncanonical A-minor motifs.
By following DSSR citations, I recently came across the article Interactive Visualization of RNA and DNA Structures by Lindow et al. The paper introduced a DNA/RNA visualization tool that integrates 1D sequence, 2D secondary structure in linear and graph representations, and 3D backbone ribbons and base ladders, all in one package. Notably, the 3D visualization was tailored for DNA/RNA structures and achieved quite impressive results. A nice feature of the 2D graph representation is the handling of multiple chains.
Reading through the main text and the supplementary material, I was surprised to see the so many locations where DSSR was mentioned, especially the following:
Our approach detects all standard and many modified nucleotides as well as the most common base pairs. Further special cases could be easily added. Yet, the system we developed should not be seen as a replacement for well established tools like DSSR. Rather, it shows what can be achieved with modern techniques in terms of both computation and rendering.
Overall, DSSR is an analysis/annotation tool that is supposedly agnostic to visualization programs. It derives a huge number of structural features that are unlikely to be matched elsewhere. I collaborated with Bob Hanson so that Jmol can directly take advantage of what DSSR has to offer, not just for the visualization of (modified) nucleotides and some common base pairs, but also the interactive selection of loops, pseudoknots, coaxial stacks, and various motifs. In particular, the SQL-like selection syntax Bob developed is really flexible and extremely powerful. I collaborated with Thomas Holder so that PyMOL can gain DNA/RNA domain knowledge. The resultant dssr_block PyMOL plugin is quite useful for creating base/base-pair block images with many revealing features, especially for small to medium-sized DNA/RNA structures. It is obvious to me that PyMOL (or any other molecular visualization tool) would benefit greatly from SQL-like selections of DSSR-derived features of nucleic acid structures, just as Jmol does.
In the Lindow et al. paper, some of the references to DSSR are technical in nature. Here, I’d like to respond and clarify each of them. Since DSSR is being actively developed and supported, I always welcome any feedback on the 3DNA Forum. Following and responding to literature represents another way that I strive to make DSSR a better tool to serve the community.
Built on their experience from 3DNA, Lu et al. developed DSSR [27], a very powerful tool to analyze RNA structures that uses Jmol for the 3D visualization. Recently, Hanson and Lu described this integration [10], which is based on a JSON-interface that directly couples DSSR and the 3D visualization of Jmol. This is a great improvement, but still missing is the integration of 2D secondary structure visualizations and brushing & linking techniques to enable simple selection with and exploration of the 3D molecular structure. One contribution of this paper is to show how a full linking between 3D and 2D visualizations can be done and what benefits arise from such a tight coupling (see Sects. 8 and 9).
This is a valid point, and the authors did a good job. Actually, one of the reviewers of our DSSR-Jmol paper brought up this point, and we acknowledged the limitation. While passing DSSR-derived secondary structural features (in DBN or .ct format) to a 2D visualization tool is straightforward, the connection would not be as smooth as we’d like it to be.
For this purpose, other approaches rely on the unique naming and ordering of the atoms [27], for example, N1, C2, N3, C4, C5, C6 etc. We found that this information is not always reliable.
The naming of the purines and pyrimidines follows the IUPAC standard and is a prerequisite of DNA/RNA structures in the PDB. In my experience, I have never found a single case where such information is not reliable. See below for abasic sites in PDB id 3BWP, and 4SU (4-thiouridine) in PDB id 5AFI.
We compared these results with the latest version of DSSR [27]. Our approach is able to correctly detect all regular nucleotides and most of the modified and undefined nucleotides. In the following, we describe the minor differences.
It is not clear what was the “latest” version of DSSR that was actually used in the paper. Note that DSSR has version info as in v1.8.3-2018oct29
. I deliberately put the release date along with the version number.
For dataset 4RGE, we detected 3 modified uracil nucleotides that were not labeled as modified by DSSR. These nucleotides have a DNA backbone instead of an RNA one.
DSSR takes A, C, G, T, U as standard nucleotides, even if T is in RNA or U is in DNA. So this result is expected.
Dataset 3BWP contains 7 nucleotides that only consist of the backbone part without bases. While our approach marks these as undefined, in DSSR they are not detected at all.
The 7 nucleotides on 3BWP are abasic sites, i.e., without base atoms (N1, C2, N3, C4, C5, C6 etc), so they do not possess base reference frames. From early on, DSSR had the --abasic
option for such cases. As of v1.7.3-2017dec26, DSSR directly incorporated abasic sites into the analysis. So thereafter they are detected by DSSR, by default.
Furthermore, in 5AFI we mark 3 nucleotides as undefined, while these are detected as a modified uracil by DSSR. This is due to the base containing sulfur instead of oxygen, so they possibly are sulfur analogs of uracil.
Presumably, the authors are referring to 4SU, 4-thiouridine, clearly a modified nucleotide occurring in 137 PDB entries (as of 2018oct28). DSSR detects three cases in 5AFI, as shown here: 4SU-u 3 v.4SU8,w.4SU8,y.4SU8
We also compared the results of our base pair detection (Suppl. Tab. 1). We determined all Watson-Crick, Hoogsteen, and Wobble pairs, and the reverse versions of the first two. For most of the datasets, our method returned the same results as DSSR. In particular, both approaches never created contradicting results, which means all common base pairs had identical pair type. In general, our geometrical approach generates slightly more base pairs compared to DSSR. However, when investigating both, the base pairs determined by DSSR but not by our approach and vice versa, we found that most of these pairs are border-line cases, where the decision was made depending on the threshold of the geometrical heuristic. Only in a few cases, the differences were not clear for both approaches, see Suppl. Fig. 3.
In Suppl. Fig. 3,
… However, the hydrogen bonds for classical G-U Wobble pairs seem to be quite unrealistic for the bottom left pair. Either this is a limitation of DSSR or it is some kind of specific Wobble pair with other hydrogen bonds than the depicted ones that our approach does not detect.
I echo the point that border-line cases could cause discrepancies between different methods. However, things can get easily clarified in concrete examples. Unfortunately, the authors did not specify the cases used in their Suppl. Fig. 3. I finally figured out the DSSR-assigned G-U Wobble pair in PDB id 1S72, U2586—G2592. As shown in the figure below, DSSR detects two H-bonds (dashed pink lines), "N3(imino)*N2(amino)[3.05],O4(carbonyl)-N1(imino)[2.77]"
. Note that one of the H-bonds is between two donors, N3(U) and N2(G), thus the * symbol. The H-bonds are by no means as those in “classical G-U Wobble pairs”. Yet, the pair is clearly Wobble-like, and that’s why it was assigned “Wobble”. To avoid such confusions, I’ve revised DSSR to tighten the criteria of G-U Wobble pair. As of v1.8.3-2018oct29, this pair is called "~Wobble"
.
![DSSR-assigned ~Wobble pair DSSR-assigned ~Wobble pair]](http://docs.x3dna.org/images/1s72-UxG-std.png)
Nevertheless, our evaluation (Sect. 8.1) shows that with the proposed approaches in terms of quality we get very similar results to the ones obtained by tools like DSSR. In terms of speed, DSSR needs much longer run times. For example, for 4U4O, DSSR needed ~15 min for the secondary and tertiary structure analysis [27], while our algorithm only needs ~0.2 s (see Tab. 1).
As noted above, DSSR provides far more structural features than just the identifications of nucleotides and several common base pairs. Even for the identified base pairs, DSSR provides many more annotations and structural parameters than just the named pairs picked by the authors. Not surprisingly, DSSR is slower than the dedicated method for a specific purpose.
As of DSSR v1.8.3-2018oct29, I’ve added the --pair-only
option that just outputs a complete listing of base-pairing information and then stops. Some sample runs are as below:
x3dna-dssr -i=1ehz.pdb --pair-only
x3dna-dssr -i=1ehz.pdb --pair-only --more
x3dna-dssr -i=1ehz.pdb --pair-only --json
x3dna-dssr -i=1ehz.pdb --pair-only --json | jq '.pairs[] | select(.name=="WC")'
x3dna-dssr -i=1ehz.pdb --pair-only --more --json | jq .
x3dna-dssr -i=4u4o.cif --pair-only -o=4u4o-pairs.txt
Compared to the default settings, DSSR runs ~10 times faster when the --pair-only
option is set; 36s vs 5m48s for 4U4O on my MacBook Pro 2017 (2.9 GHz Intel Core i7). Note the timing here is a complete run of the DSSR program (as shown above), from reading the mmCIF file to writing out all the derived features. In my hand, simply reading and parsing the 85MB 4U4O.cif
would take ~5s. As a reference, just loading 4U4O.cif
into PyMOL takes >6s. I’m thus more than surprised (and remain to be convinced) by the claim that their new algorithm “only needs” ~0.2s “for the secondary and tertiary structure analysis” of 4U4O.
Via Google Scholar, I noticed the recent publication in Nucleic Acids Research by Meier et al. titled Structure and hydrodynamics of a DNA G-quadruplex with a cytosine bulge. Reading through the article, I am pleased to see the section “Nucleic acid geometry and visualization” under MATERIALS AND METHODS:
We used the program DSSR (53) of the 3DNA suite (54) to analyse the nucleic acid backbone and the base pair geometry from the 3D structures. We reported the ‘simple’ base-pair parameters for buckle, propeller twist and stagger which are more intuitive for non-canonical base-pairs than the classic base-pair parameters as explained in the program manual and the 3DNA website (http://home.x3dna.org/highlights/details-on-the-simple-base-pair-parameters, http://home.x3dna.org/articles/simple-parameters-for-non-Watson-Crick-base-pairs). We wrote an R (55) script that automatically creates a backbone angle plot from the output of the DSSR program. The script can be downloaded from the 3DNA forum at http://home.x3dna.org. The nucleic acid was visualized in PyMOL and the dssr block plugin (The PyMOL Molecular Graphics Sys- tem, Version 2.0, Schro ̈dinger, LLC, https://pymol.org/). …
This is the first time (I’m aware of) that the ‘simple’ base-pair parameters introduced in 3DNA v2.3 is cited in a peer-reviewed journal article. I’m also glad to know that the blog posts on the 3DNA homepage are read, and even referenced in a publication — which surely will prompt me to write more. This is also the first time that the dssr_block PyMOL plugin is cited. I must say that Figures 1, 5, and 6 from the paper look gorgeous. Among other things, the G-tetrads and the surrounding base identity are immediately obvious using the simple color code: A, red; T, blue, C, yellow, and G, green. See Fig. 1 below.
![DSSR-enabled block images [Fig. 1 of Meier18-nar-gky307] DSSR-enabled block images [Fig. 1 of Meier18-nar-gky307]](http://docs.x3dna.org/images/Meier18-nar-gky307fig1.jpeg)
In the section on “DATA AVAILABILITY”, the authors further noted:
Our R (55) script that automatically creates backbone angle plots from the output of the DSSR program can be downloaded from the 3DNA forum at http://home.x3dna.org.
I communicated with Markus Meier (the lead author) on the 3DNA Forum, on the thread DSSR: Analyzing NMR structures – overwritten output files. Checking the thread right now, I found that the R script (backbone_torsions_plot-1.0.tar.bz2
) has been downloaded 263 times. I appreciate Markus’s effort in contributing the R script with a working example to the DSSR user community. It has always been my hope that more DSSR users would share their scripts and examples via the 3DNA Forum.
As a side note, I met Markus in Los Angles at the 60th Annual Meeting. It was a nice experience chatting with him, and had a lunch together. We’ve kept in touch following the meeting.
I recently came across an article titled DNAproDB: an interactive tool for structural analysis of DNA-protein complexes by Sagendorf et al. in Nucleic Acids Research (NAR). Notably, the DNAproDB tool allows users to search the underlying database by combining features of the DNA, protein, or DNA-protein interactions at the interface. Compared to the well-established NUCPLOT tool which generates only ‘static’ schematic diagrams of protein-nucleic acid interactions, DNAproDB is interactive and more user friendly, with many new features.
It was a pleasant surprise to notice that SNAP was cited in the DNAproDB NAR paper, as follows:
Nucleotide-residue interaction geometry (stacking, pseudo-pairing or other) is determined using SNAP, a new component of the 3DNA program suite (35). SNAP also serves as a fall-back for calculating hydrogen bonds if HBPLUS cannot process the file.
I am glad that SNAP has also been used for identifying H-bonds where HBPLUS fails. The H-bonding detection algorithm, initially implemented in 3DNA (v2.3 and before) and refined in DSSR/SNAP, was originally intended to make the 3DNA software fully independent of third-party tools. I did not expect this feature could one day compete with dedicated H-bond finding tools, such as HBPLUS.
By the way, 3DNA is also cited in the DNAproDB NAR paper, as below:
DNA base pairing, shape parameters and conformation are derived from the 3DNA program suite (29) with a 10.0 Å cut-off for helix breaking.
While browsing the latest issue (May 2017) of the RNA journal, I came across the paper titled The structure of an E. coli tRNAfMet A1–U72 variant shows an unusual conformation of the A1–U72 base pair by Monestier et al.. Reading through the text, I am pleasantly surprised by the two references to DSSR as shown below:
An analysis using DSSR (Lu et al. 2015) identifies all the secondary structure elements characteristic of the classical cloverleaf secondary structure as well as usual tertiary interactions that stabilize the L-shaped tertiary fold of the molecule.
As a consequence, the opening parameter (Lu et al. 2015) of the A1–U72 base pair becomes unusually high (153.42°). The NH2 group of A1 points toward the minor groove of the acceptor helix. An interaction between the N1 of A1 and the O2 of U72 (d = 3.0 Å) is observed which requires protonation of the N1 atom of A1.
The PDB id for the deposited structure is 5l4o. Running DSSR on this structure is straightforward: x3dna-dssr -i=5l4o.pdb --more
. As with the classic yeast phenylalanine tRNA (PDB id: 1ehz), DSSR identifies two helices, three hairpin loops, one [2,1,5,0] four-way junction loop, among other features.
With regard to the unusual A1-U72 pair highlighted in the title of the paper, DSSR provides the following information. Note the *
in the unconventional N1*O2
H-bond.
1 A.A1 A.U72 A+U -- n/a tWW tW+W
[-14.4(...) ~C3'-endo lambda=32.9] [-172.4(anti) ~C3'-endo lambda=65.0]
d(C1'-C1')=10.80 d(N1-N9)=9.19 d(C6-C8)=10.68 tor(C1'-N1-N9-C1')=173.6
H-bonds[1]: "N1*O2(carbonyl)[2.99]"
interBase-angle=6 Simple-bpParams: Shear=3.53 Stretch=1.71 Buckle=2.0 Propeller=-6.0
bp-pars: [-0.32 3.91 0.01 6.32 -0.26 153.56]
This citation is yet another example of DSSR’s adoption by experimental biologists. I can only expect to see more such type of DSSR usages in the coming years.
Recently, I came across the article URS DataBase: universe of RNA structures and their motifs by Baulin et al. in Database, an online journal of biological databases and curation. I am glad to see that DSSR is used in the URSDB, as quoted below.
In the “Input data” subsection of “Materials and methods”:
RNA-containing structures were extracted from the PDB in mmCIF format; each file was divided into models. The base pairs (both canonical and non-canonical) and dinucleotide steps were annotated using the DSSR program from 3DNA toolkit (26). We also exploited detailed information provided by DSSR on given elements such as geometric parameters, types according to different classifications and various details on base conformations.
Moreover, under “Future development”, the authors said:
We plan to perform a comparative analysis of programs that annotate base pairs in RNA-containing PDB files. We will consider the four most popular programs, FR3D (35), MC-Annotate (36), RNAView (37) and DSSR (26). According to the analysis the annotation of the base pairs will be refined. In addition, we plan to include in the database annotations of base-phosphate, base-ribose and base stacking contacts and to implement search of such data.
It is gratifying to see DSSR listed as one of “the four most popular programs” for annotating RNA base pairs. It’d also be interesting to see how DSSR compares with FR3D, MC-Annotate, and RNAView from the user’s perspective.