It gives me great pleasure to announce that the 3DNA/DSSR project is now funded by the NIH R24GM153869 grant, titled "X3DNA-DSSR: a resource for structural bioinformatics of nucleic acids". I am deeply grateful for the opportunity to continue working on a project that has basically defined who I am. It was a tough time during the funding gap over the past few years. Nevertheless, I have experienced and learned a lot, and witnessed miracles enabled by enthusiastic users.
Since late 2020 when I lost my R01 grant, DSSR has been licensed by the Columbia Technology Ventures (CTV). I appreciate the numerous users (including big pharma) who purchased a DSSR Pro License or a DSSR Basic paid License. Thanks to the NIH R24GM153869 grant, we are pleased to provide DSSR Basic free of charge to the academic community. Academic Users may submit a license request for DSSR Basic or DSSR Pro by clicking "Express Licensing" on the CTV landing page. Commercial users may inquire about pricing and licensing terms by emailing techtransfer@columbia.edu, copying xiangjun@x3dna.org.
The current version of DSSR is v2.4.5-2024sep24 which contains miscellaneous bug fixes (e.g., chain id with > 4 chars) and minor improvements. This release synchronizes with the new R24 funding, which will bring the project to the next level. All existing users are encouraged to upgrade their installation.
Lots of exciting things will happen for the project. The first thing is to make DSSR freely accessible to the academic community. In the past couple of weeks, CTV have already issued quite a few DSSR Basic Academic licenses to users from all over the world. So the demand is high, and it will become stronger as more academic users become aware of DSSR. I'm closely monitoring the 3DNA Forum, and is always ready to answer users questions.
I am committed to making DSSR a brand that stands for quality and value. By virtue of its unmatched functionality, usability, and support, DSSR saves users a substantial amount of time and effort when compared to other options. My track record throughout the years has unambiguously demonstrated my dedication to this solid software product.
DSSR Basic contains all features described in the three DSSR-related papers, and includes the originally separate SNAP program (still unpublished) for analyzing DNA/RNA-protein complexes. The Pro version integrates the classic 3DNA functionality, plus advanced modeling routines, with email/Zoom/phone support.
A starting structure of suitable sequence is a prerequisite for many applications, including downstream use in X-ray crystallography, NMR, and molecular dynamics (MD) simulations. Browsing through the literature, I’ve noticed the following tools for such a purpose.
- The make-na server, a web-based automated tool for making nucleic acid helices powered by NAB. It supports abasic sites via the underscore character (
_
). According to the help page, “The structure file represents the abasic as the 3-letter code ‘3DR’ in DNA strands and ‘ N’ in RNA strands. These are Protein Data Bank conventions.” An example input is shown below:
ATACCGATACG_TAGAC
TG_CTATGCTATCTGT_
- The NAB itself, and the standalone fd_helix.c program which supports 6 fiber-based models of DNA or RNA.
- The NUCGEN program from the Bansal group. “The NUCGEN software generates double helical models with the backbone fixed in B-form DNA, but with appropriate modifications in the input data, it can also generate A-form DNA and RNA duplex structures.”
- 3DNA and its web interface. The ‘rebuild’ program can be used for constructing customized, single or duplex DNA/RNA structures based on a set of base-pair and step (helical) parameters. Moreover, the sugar-phosphate backbone in A-, B- or RNA conformation is allowed. The ‘fiber’ program incorporates a comprehensive list of 56 regular models, based mostly on fiber diffraction data. The list includes single, duplex, triplex, quadruplex, DNA, RNA structures or their hybrids. Notable, the classic Pauling’s triplex model is also available. The 3DNA web 2.0 makes these model-building features readily accessible to a large user base.
Overall, each of the tools listed above has its unique features and may fit better for different applications. It is to the benefit of the user community to have a choice.

Nowadays, I’ve been used to google searches as a quick way to solve problems. Once in a while, I come across a tip or trick that fixes an issue at hand and then move on. However, I may late on meet a similar problem, but only vaguely remember how I solved it previously. So I’d need to start googling around again. This list is a remedy for such situations, and it will be continuously updated. While the list is created for my own reference, it may also be useful to other viewers of the post, presumably reaching here via google.
icdiff
— show diff with color
scc
— strip C comments
Taskwarrior (taks)
— manage TODO list from terminal
httpie
as a replacement of curl
and wget
byebug
and pry
for debugging Ruby
ag
to search for PATTERN in source files, replacing grep
fd
to find files and directories
bat
to view files with syntax highlighting (in place of cat
)
exa
as an alternative to ls
bench
to benchmark code
asciinema
and svg-term
to record terminal activity as an SVG animation. Another option is termtosvg
. Moreover, the trio ttyrec, ttyplay, ttygif
can record, play terminal screen recordings, and convert it into smooth GIF
wrk
to benchmark HTTP APIs
hub
— git wrapper for GitHub
tail -n +2
to skip the first line (starting from the second line)
sudo -i -u user_id
, the -i
or --login
option invokes login shell
- Understanding Shell Script’s idiom: 2>&1 — redirect ‘stderr’ to ‘stdout’ via ’2>&1’ in
bash
shell.
- Ruby one-liners
ruby -pi.bak -e "gsub(/SOME_PATTERN/, 'other_text')" files
for global replacement of SOME_PATTERN by other_text in files
ruby -pe 'gsub(/_/, ".")'
globally replace ‘_’ with ‘.’

Nucleic acids structural bioinformatics starts with the identification of nucleotides (nts) from atomic coordinates. As biopolymers, RNA and DNA have standard IUPAC names of atoms for the five bases (see the Figure below), sugars (ending with prime, e.g., C1’, O2’), and the phosphate (P, OP1, and OP2). The atomic coordinates (in PDB or mmCIF format) from the Protein Data Bank (PDB) follow the convention.
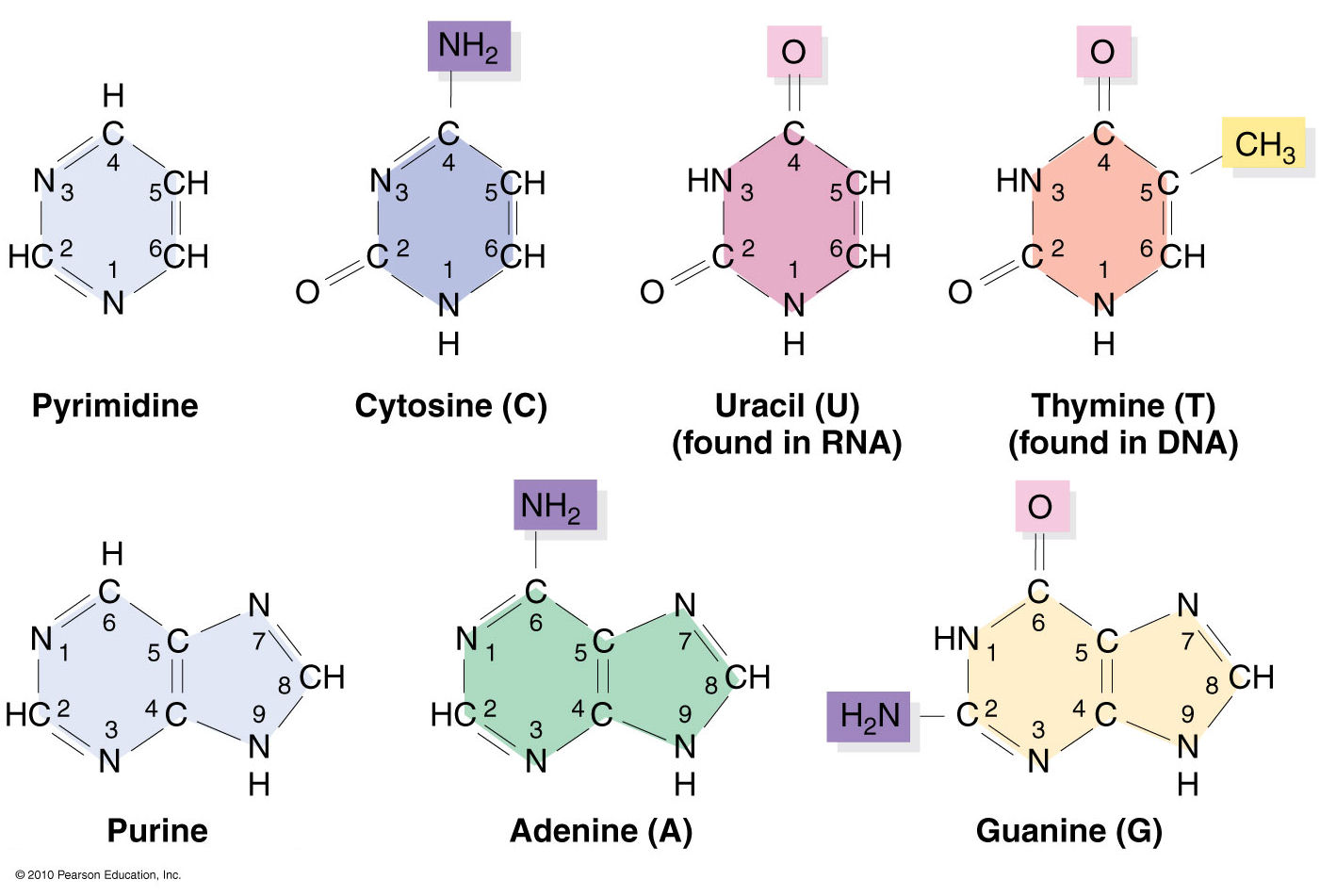
Trained as a chemist, I am aware that the bases are aromatic, heterocyclic compounds (purines and pyrimidines). Moreover, the five standard bases (A, C, G, T, and U) also share a six-membered ring, with atoms named consecutively (N1, C2, N3, C4, C5, C6). This special feature can be employed to identify nts automatically, from PDB atomic coordinates. The ring skeleton is not influenced by protonation states, tautomeric forms, or modifications in base, sugar or phosphate. Early versions of 3DNA (up to v2.0) used only N1, C2, and C6 atoms to identify an nt: an additional N9 as purine, otherwise as pyrimidine. In 3DNA v2.3 and DSSR, the procedure has been refined to take advantage of all available rings atoms. It is thus more robust against distortions and still works even when any of the N1, C2, C6, or N9 atoms are mutated or missing. This blog post provides further technical details on how the method works.
The template used to identify nts is a purine, with nine base ring atoms. Purine is chosen since it contains atoms of the six-membered ring and N7, C8, and N9. Its atomic coordinates in PDB format are shown below. The coordinates are taken from ‘G’ in the standard reference frame ($X3DNA/config/Atomic_G.pdb
). Using ‘A’ as reference won’t make any difference since the RMSD between them is only 0.038 Å.
ATOM 1 N9 G A 1 -1.289 4.551 0.000 1.00 0.00 N
ATOM 2 C8 G A 1 0.023 4.962 0.000 1.00 0.00 C
ATOM 3 N7 G A 1 0.870 3.969 0.000 1.00 0.00 N
ATOM 4 C5 G A 1 0.071 2.833 0.000 1.00 0.00 C
ATOM 5 C6 G A 1 0.424 1.460 0.000 1.00 0.00 C
ATOM 6 N1 G A 1 -0.700 0.641 0.000 1.00 0.00 N
ATOM 7 C2 G A 1 -1.999 1.087 0.000 1.00 0.00 C
ATOM 8 N3 G A 1 -2.342 2.364 0.001 1.00 0.00 N
ATOM 9 C4 G A 1 -1.265 3.177 0.000 1.00 0.00 C
The nt-identification process begins with a mapping of at least three atoms in the purine, followed by a least-squares fit between corresponding atoms. For the five standard bases and most modified ones, the RMSD is normally less than 0.12 Å, as seen in the Figure below. Even the unsaturated dihydrouridine in tRNA has an RMSD of less than 0.25 Å: for the yeast phenylalanine tRNA (PDB id: e1ehz), for example, it is 0.205 Å for H2U-16, and 0.226 Å for H2U-17. DSSR uses a cutoff of 0.28 Å, covering essentially all nucleotides in the PDB. As an extreme case, the DA1 residue on chain T of PDB id 4ki4 has only three base atoms: N7, C8, and N9 (i.e., no atoms from the six-membered ring). With an RMSD of only 0.005 Å, DSSR still takes it as an nt, properly assigned as ‘A’.
Molecular dynamics (MD) simulations sometimes produce heavily distorted bases, which is over the default cutoff. Users may change the cutoff to a larger value to accommodate such unusual cases.
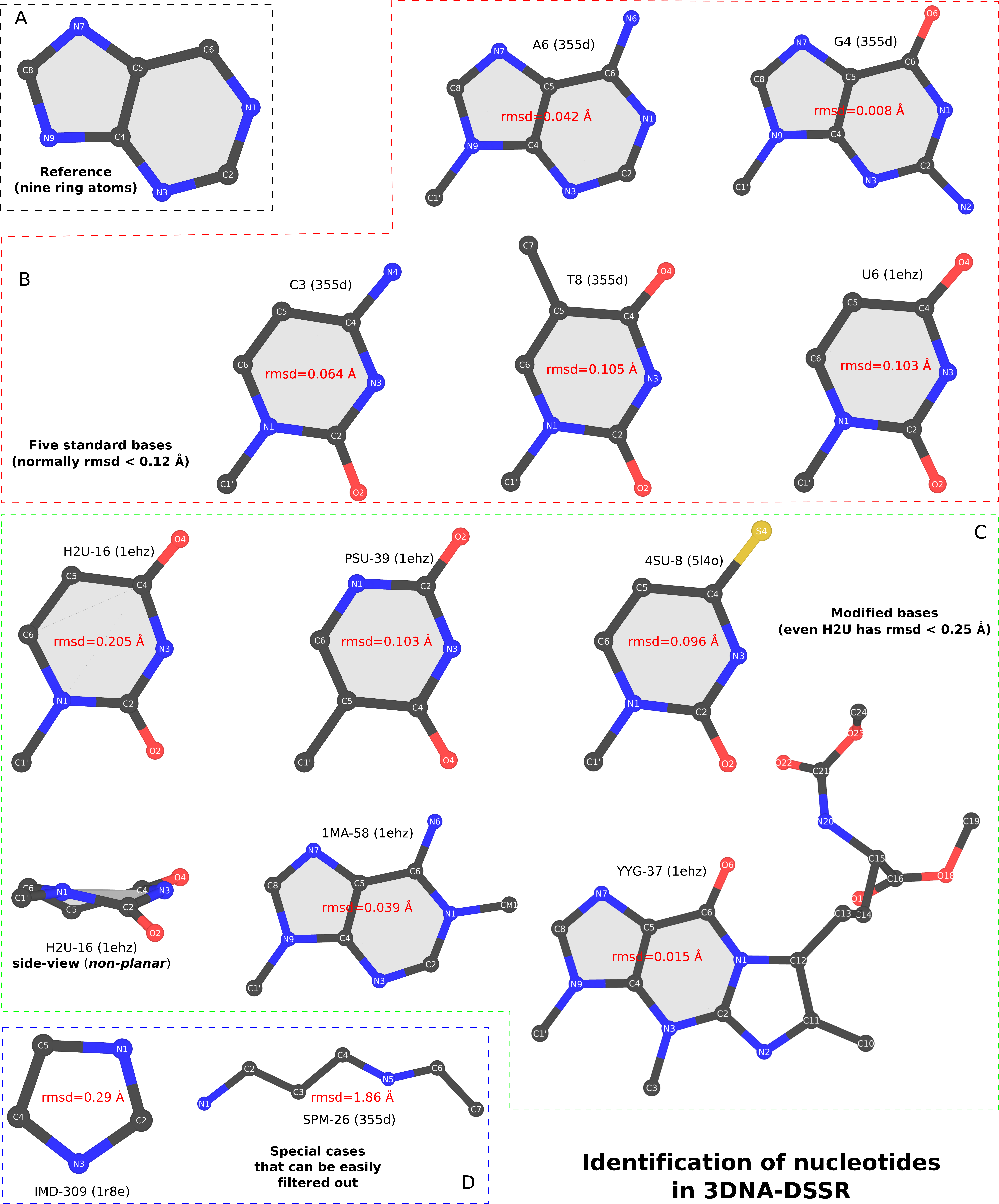
In addition to dihydrouridine, the above Figure also shows pseudouridine (PSU), 1-methyladenosine (1MA), 4-thiouridine (4SU), and the heavily modified YYG in tRNA. They are all easily identified using the same scheme. Since the nt-identification method concentrates on base rings, modifications in sugar or the phosphate group do not pose any problem. For example, in tRNA 1ehz, DSSR also identifies O2’-methylguanosine (OMG) and O2’-methylcytidine (OMC) as modified nts.
Two special cases worth mentioning. The ligand IMD in PDB id 1r8e has a five-membered ring. Its atoms are named similarly to those of an nt, and the fitted RMSD is only 0.29 Å. IMD can be filtered out by its missing of the C6 atom and having an N1—C5 covalent bond. The ligand SPM in PDB id 355d is a linear molecule, and its RMSD (1.86 Å) is clearly far off to be taken as an nt.
Another particular case (of a different kind) is the abasic sites, especially in X-ray crystal structures in the PDB. By definition, abasic sites do not have base atoms available. Thus the described method is not applicable to their characterization as nts. As of v1.7.3-2017dec26, however, DSSR has also incorporated abasic sites into the analysis pipeline, by default. The program checks backbone linkage and residue name for appropriate nt assignment. The abasic sites could constitute part of (internal) loops which would otherwise be broken into segments by DSSR.
Overall, I feel confident to say that 3DNA-DSSR has practically solved the problem of identifying nts from atomic coordinates. The method detailed herein (and outlined in the DSSR paper) is simple and easy to understand/implement. Moreover, it has been extensively tested in real-world applications for well over a decade. I’ve yet to find a single case where it does not work as expected.

Recently, a 3DNA user asked on the Forum about how to perform mutations to 3-methyladenine. The user reported that the procedure described in the FAQ entry How can I mutate cytosine to 5-methylcytosine did not work for the case of 3-methyladenine. This ‘limitation’ is easily understandable: the 3DNA mutate_bases
program must have knowledge of the target base, 3-methyladenine, to perform the mutation properly. The program works for the most common 5-methylcytosine mutations since the corresponding 5MC file (Atomic_5MC.pdb
, in the standard base-reference frame) is already included within the 3DNA distribution. By supplying a similar file for the target base, mutate_bases
runs the same for mutations to 5-methylcytosine (or other bases). This blog post outlines the procedure, using 3-methyladenine as an example.
A ligand name search for 5-methylcytosine on the RCSB PDB led to only two matched entries: 2X6F and 3MAG. The ligand id is 3MA. Since 3MAG has a better resolution (1.8 Å) than 2X6F (3.3 Å), its 3MA ligand was extracted from the corresponding PDB file (3MAG.pdb
). The atomic coordinates, excluding those for the two hydrogens, are as below. Note that the 3-methyl carbon atom is named CN3
.
HETATM 2960 N9 3MA A 600 16.587 14.258 22.170 1.00 49.87 N
HETATM 2961 C4 3MA A 600 17.123 13.100 21.622 1.00 50.46 C
HETATM 2962 N3 3MA A 600 16.877 11.811 22.009 1.00 50.37 N
HETATM 2963 CN3 3MA A 600 15.983 11.363 23.063 1.00 50.41 C
HETATM 2964 C2 3MA A 600 17.590 10.968 21.241 1.00 50.11 C
HETATM 2965 N1 3MA A 600 18.422 11.217 20.224 1.00 49.27 N
HETATM 2966 C6 3MA A 600 18.627 12.484 19.858 1.00 48.99 C
HETATM 2967 N6 3MA A 600 19.426 12.709 18.829 1.00 46.12 N
HETATM 2968 C5 3MA A 600 17.949 13.503 20.593 1.00 49.89 C
HETATM 2969 N7 3MA A 600 17.929 14.900 20.488 1.00 49.84 N
HETATM 2970 C8 3MA A 600 17.113 15.286 21.434 1.00 49.58 C
After running the 3DNA utility program std_base
with options -fit -A
, the corresponding atomic coordinates of 3MA are transformed to the standard base reference frame of adenine. The file must be named Atomic_3MA.pdb
, and it has the following contents:
HETATM 1 N9 3MA A 1 -1.287 4.521 0.006 1.00 49.87 N
HETATM 2 C4 3MA A 1 -1.262 3.133 0.004 1.00 50.46 C
HETATM 3 N3 3MA A 1 -2.337 2.286 -0.009 1.00 50.37 N
HETATM 4 CN3 3MA A 1 -3.743 2.648 -0.047 1.00 50.41 C
HETATM 5 C2 3MA A 1 -1.905 1.013 0.001 1.00 50.11 C
HETATM 6 N1 3MA A 1 -0.662 0.520 0.004 1.00 49.27 N
HETATM 7 C6 3MA A 1 0.366 1.372 -0.003 1.00 48.99 C
HETATM 8 N6 3MA A 1 1.588 0.867 -0.034 1.00 46.12 N
HETATM 9 C5 3MA A 1 0.068 2.768 0.003 1.00 49.89 C
HETATM 10 N7 3MA A 1 0.875 3.914 -0.003 1.00 49.84 N
HETATM 11 C8 3MA A 1 0.026 4.909 -0.003 1.00 49.58 C
Note that in file Atomic_3MA.pdb
, (1) the z-coordinates of the base atoms are close to zeros, (2) the ordering of atoms is as in the original ligand of 3MA shown above.
With Atomic_3MA.pdb
in place (in the current working directory, or the $X3DNA/config
folder), one can perform 3-methyladenine mutations using mutate_bases
. For illustration purpose, let’s generate a B-form DNA with base sequence GACATGATTGCC using the 3DNA fiber
program:
fiber -seq=GACATGATTGCC fiber-BDNA.pdb
To mutate A7 to 3MA, one needs to run mutate_bases
as following:
mutate_bases "chain=A s=7 m=3MA" fiber-BDNA.pdb fiber-BDNA-A7to3MA.pdb
The result of the mutation is shown in the figure below. Note that the backbone has identical geometry as that before the mutation, and the mutated 3MA-T pair has exactly the same parameters (propeller/buckle etc) as the original A-T. These are the two defining features of the 3DNA mutate_bases
program.
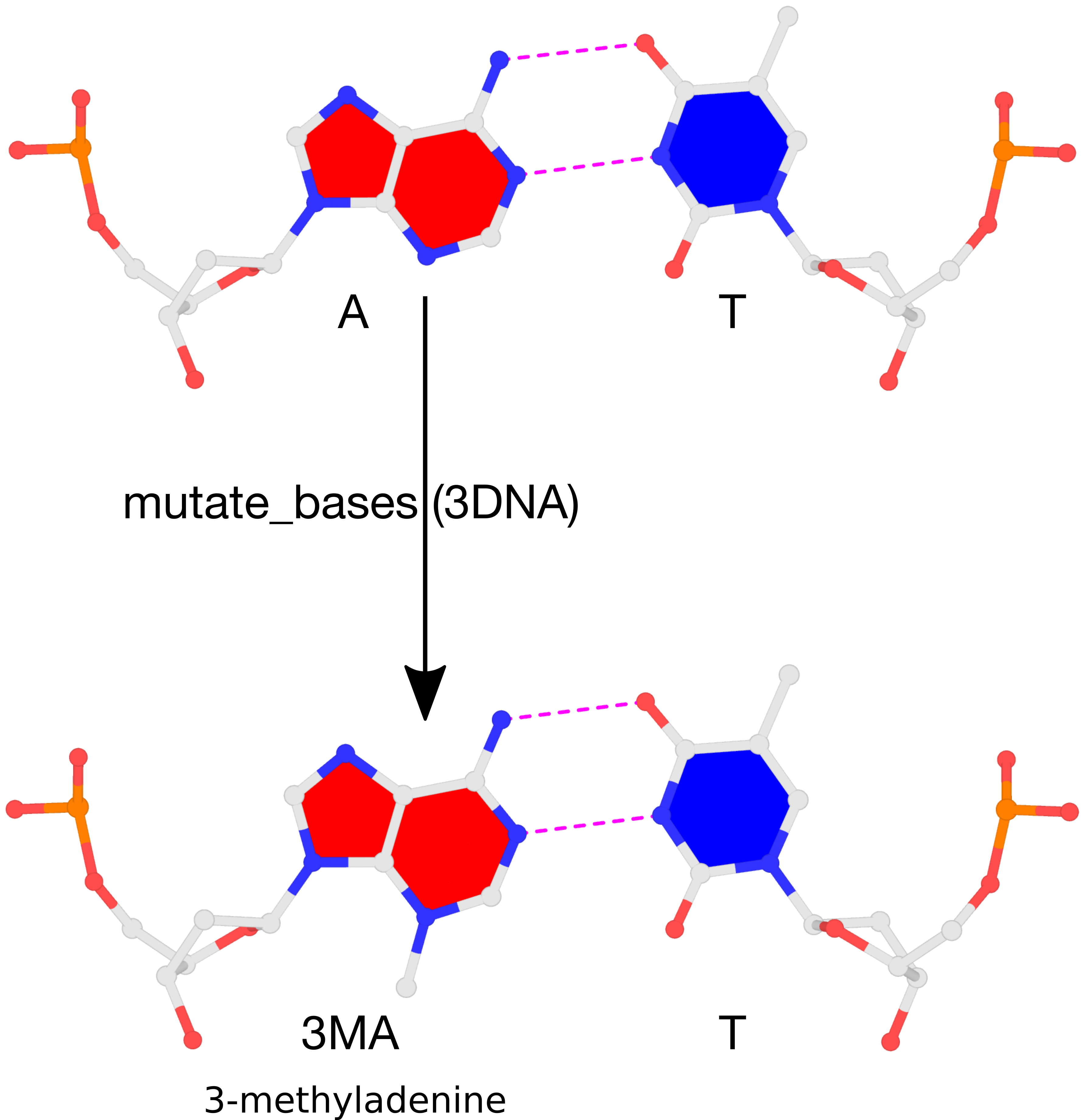
Please see the thread mutations to 3-methyladenine on the 3DNA Forum to download files fiber-BDNA.pdb
and fiber-BDNA-A7to3MA.pdb
.

Over the past couple of months, I’ve further enhanced the DSSR-derived structural features for Q-quadruplexes (G4). One was the implementation of the single descriptor of intramolecular canonical G4 structures with three connecting loops recently proposed by Dvorkin et al. The descriptor contains the number of guanines in the G4 stem, the type and relative direction of loops linking G-tracts of the stem, and the groove-widths associated with lateral loops. For example, PDB entry 2GKU (see the DSSR-enabled PyMOL schematic image below, Fig. 1A) has the following DSSR output.
List of 1 G4-stem
Note: a G4-stem is defined as a G4-helix with backbone connectivity.
Bulges are also allowed along each of the four strands.
stem#1[#1] layers=3 INTRA-molecular loops=3 descriptor=3(-P-Lw-Ln) note=hybrid-1(3+1) UUDU anti-parallel
1 glyco-bond=ss-s groove=-wn- mm(<>,outward) area=14.24 rise=3.58 twist=16.8 nts=4 GGGG A.DG3,A.DG9,A.DG17,A.DG21
2 glyco-bond=--s- groove=-wn- pm(>>,forward) area=13.12 rise=3.71 twist=25.9 nts=4 GGGG A.DG4,A.DG10,A.DG16,A.DG22
3 glyco-bond=--s- groove=-wn- nts=4 GGGG A.DG5,A.DG11,A.DG15,A.DG23
strand#1 U DNA glyco-bond=s-- nts=3 GGG A.DG3,A.DG4,A.DG5
strand#2 U DNA glyco-bond=s-- nts=3 GGG A.DG9,A.DG10,A.DG11
strand#3 D DNA glyco-bond=-ss nts=3 GGG A.DG17,A.DG16,A.DG15
strand#4 U DNA glyco-bond=s-- nts=3 GGG A.DG21,A.DG22,A.DG23
loop#1 type=propeller strands=[#1,#2] nts=3 TTA A.DT6,A.DT7,A.DA8
loop#2 type=lateral strands=[#2,#3] nts=3 TTA A.DT12,A.DT13,A.DA14
loop#3 type=lateral strands=[#3,#4] nts=3 TTA A.DT18,A.DT19,A.DA20
The descriptor=3(-P-Lw-Ln) means that the G4 structure has three layers of G-tetrads, connected via three loops: the first is the Propeller loop in anti-clockwise (negative) direction, then the Lateral loop passing a wide groove anti-clockwise, and finally another Lateral loop passing a narrow groove, also anti-clockwise. The DSSR symbols follow those of Dvorkin et al. but with capital letters L, P, and D for lateral, propeller, and diagonal loops instead of lower case letters (l, p, d) to avoid using subscript for groove-width info. So the 2GKU descriptor 3(-P-Lw-Ln) from DSSR corresponds to 3(-p-lw-ln) of Dvorkin et al.
The DSSR-enabled, PyMOL-rendered, block image in Fig. 1A makes the three G-tetrad layers (squared green blocks) immediately obvious. Other base identities and stacking interactions also become clear — for example, the A24 (in red) stacks on the top G-tetrad, and T1-A20 pair stacks with the bottom G-tetrad.
Two other PDB entries (2LOD and 2KOW) are illustrated in Fig. 1B and Fig. 1C. They have different topologies than 2GKU (Fig. 1A). DSSR is able to characterize all of them consistently.
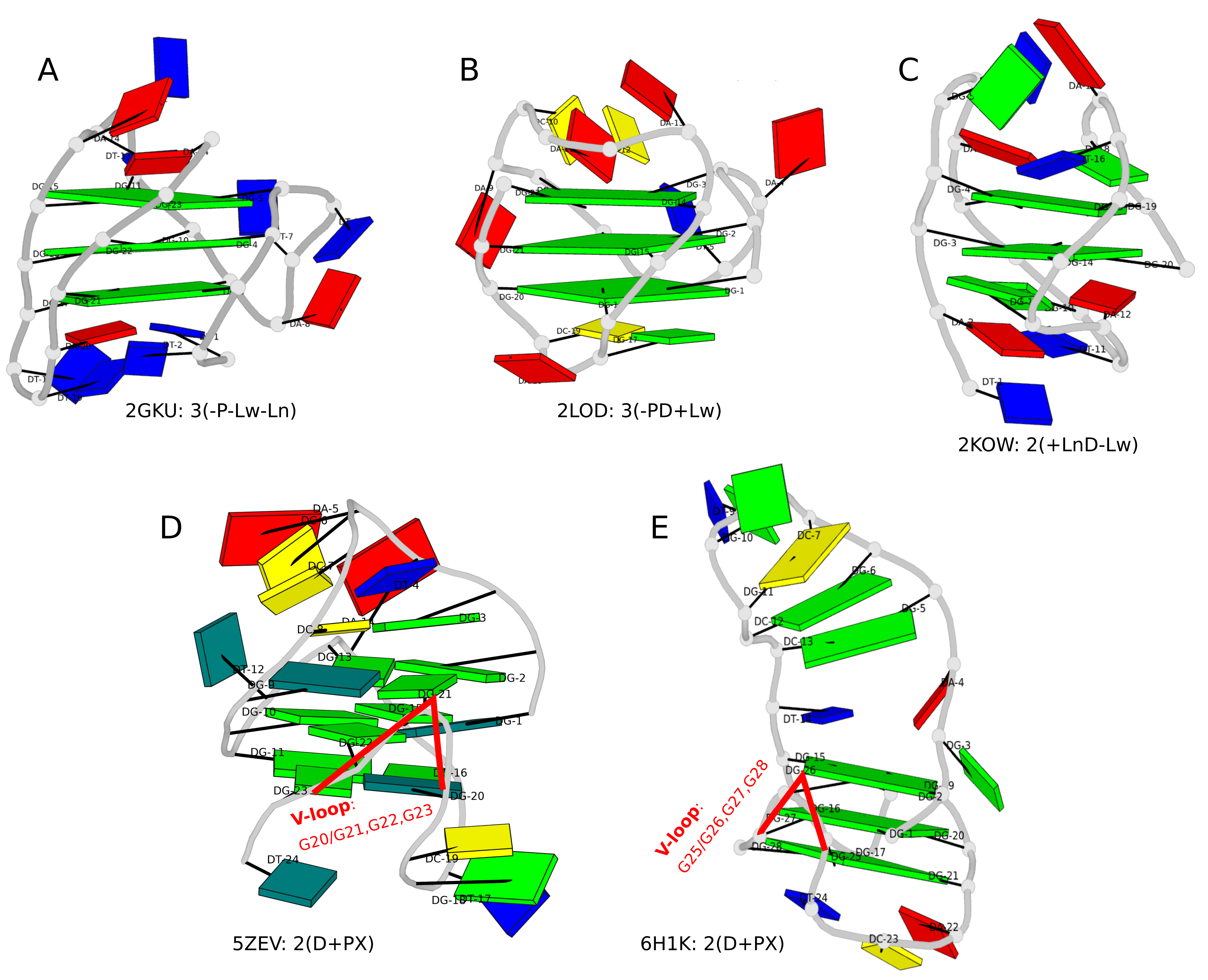
Figure 1. DSSR-enabled, PyMOL-rendered, block images of five G-quadruplexes. A in red, C in yellow, G (and G-tetrad) in green, and T in blue.
Another G4-related new feature in DSSR is the detection of V-shaped loops in noncanonical G4 structures where one of the four G-G columns (strands) that link adjacent G-tetrads is broken. Two of recent PDB examples with V-loops are shown in Fig. 1D (5ZEV) and Fig. 1E (6H1K). An excerpt of DSSR output for the PDB entry 6H1K is shown below.
List of 1 G4-helix
Note: a G4-helix is defined by stacking interactions of G4-tetrads, regardless
of backbone connectivity, and may contain more than one G4-stem.
helix#1[1] stems=[#1] layers=3 INTRA-molecular
1 glyco-bond=-sss groove=w--n mm(<>,outward) area=12.76 rise=3.47 twist=18.2 nts=4 GGGG A.DG2,A.DG19,A.DG15,A.DG26
2 glyco-bond=s--- groove=w--n pm(>>,forward) area=12.84 rise=3.07 twist=33.4 nts=4 GGGG A.DG1,A.DG20,A.DG16,A.DG27
3 glyco-bond=s--- groove=w--n nts=4 GGGG A.DG25,A.DG21,A.DG17,A.DG28
strand#1 DNA glyco-bond=-ss nts=3 GGG A.DG2,A.DG1,A.DG25
strand#2 DNA glyco-bond=s-- nts=3 GGG A.DG19,A.DG20,A.DG21
strand#3 DNA glyco-bond=s-- nts=3 GGG A.DG15,A.DG16,A.DG17
strand#4 DNA glyco-bond=s-- nts=3 GGG A.DG26,A.DG27,A.DG28
****************************************************************************
List of 1 G4-stem
Note: a G4-stem is defined as a G4-helix with backbone connectivity.
Bulges are also allowed along each of the four strands.
stem#1[#1] layers=2 INTRA-molecular loops=3 descriptor=2(D+PX) note=UD3(1+3) UDDD anti-parallel
1 glyco-bond=s--- groove=w--n mm(<>,outward) area=12.76 rise=3.47 twist=18.2 nts=4 GGGG A.DG1,A.DG20,A.DG16,A.DG27
2 glyco-bond=-sss groove=w--n nts=4 GGGG A.DG2,A.DG19,A.DG15,A.DG26
strand#1 U DNA glyco-bond=s- nts=2 GG A.DG1,A.DG2
strand#2 D DNA glyco-bond=-s nts=2 GG A.DG20,A.DG19
strand#3 D DNA glyco-bond=-s nts=2 GG A.DG16,A.DG15
strand#4 D DNA glyco-bond=-s nts=2 GG A.DG27,A.DG26
loop#1 type=diagonal strands=[#1,#3] nts=12 GAGGCGTGGCCT A.DG3,A.DA4,A.DG5,A.DG6,A.DC7,A.DG8,A.DT9,A.DG10,A.DG11,A.DC12,A.DC13,A.DT14
loop#2 type=propeller strands=[#3,#2] nts=2 GC A.DG17,A.DC18
loop#3 type=diag-prop strands=[#2,#4] nts=5 GACTG A.DG21,A.DA22,A.DC23,A.DT24,A.DG25
****************************************************************************
List of 2 non-stem G4 loops (INCLUDING the two terminal nts)
1 type=lateral helix=#1 nts=5 GACTG A.DG21,A.DA22,A.DC23,A.DT24,A.DG25
2 type=V-shaped helix=#1 nts=4 GGGG A.DG25,A.DG26,A.DG27,A.DG28
Note that here a new loop type (diag-prop
) and topology description symbol (X
) are introduced. In developing DSSR in general, and G4-related features in particular, I’ve always tried to follow conventions widely used by the community. Whereas inconsistency exists, I pick up the ones that are in line with other parts of DSSR. For unique DSSR features lacking outside references, I came up my own nomenclature. When DSSR becomes more widely used, it may serve to standardize G4 nomenclatures.

From early on, the --json
and --nmr
options in DSSR have provided a convenient means to analyze an ensemble of solution NMR structures in the standard PDB/mmCIF format, as those available from the Protein Data Bank (PDB). The usage is very simple, as shown below for the PDB entry 2lod. The parameters for each model can be easily parsed from the output JSON stream.
x3dna-dssr -i=2lod.pdb --nmr --json
A practical example of the DSSR JSON/NMR usage for the analysis of RNA backbone torsion angles can be found on the 3DNA Forum.
While not a practitioner of molecular dynamics (MD) simulations, I’ve regularly followed the relevant literature. I know of the popular tools such as MDanalysis, MDTraj, and CPPTRAJ that are dedicated to analyze trajectories of MD simulations. I understand the subtleties MD may have, and I’m also sure of the unique features DSSR has to offer. By design, I made the DSSR interface to MD straightforward, by simply following commonly-used standard data formats: the MODEL/ENDMDL delineated PDB (or the PDBx/mmCIF) format for input, and JSON for output. Overall, I had expected that DSSR would complement the dedicated tools (e.g., MDanalysis, MDTraj, and CPPTRAJ) for MD analysis.
Over the years, DSSR has gradually gained recognition in the MD field. At a meeting, I once heard of a user complaining that DSSR is too slow for the analysis of millions of frames of MD simulations. Yet, without access to a large MD dataset and direct collaborations from a user, the speed issue could not be pursued further. In my experience, I knew DSSR is fast enough for the analysis of NMR ensembles from the PDB. This situation has completely changed recently, after a user reported on the 3DNA Forum on the slowness of DSSR on MD analysis.
Do you have an idea why the backbone parameter for a nucleic acids are so much faster calculated with do_x3dna
than with DSSR? Analyzing a trajectory with 100k frames take for a native structure approx. 2 hours with do_x3dna. A native RNA structure with DSSR will take approx. 10 days (10k frames/day). I need to run DSSR, because my system contains an abasic site.
With the above and follow-up information provided, I was able to fix the DSSR algorithm for parsing MD trajectories, among other things. Now DSSR reads a trajectory sequentially frame-by-frame at constant speed. The same 100K frames takes 36 minutes to finish instead of 10 days, which is an increase of 10*24*60/36=400 times. This 100x speedup was later on verified when I tested DSSR on the 1000-structure trajectory the user supplied.
So as of v1.7.8-2018sep01, DSSR is quick enough for real-world applications on MD analysis. In the releases of DSSR afterwards, I’ve further polished the code and added some new features. All things considered, DSSR is bound to become more relevant in the active MD field in the years to come.
By the way, for those who do not like the --nmr
option, --md
or --ensemble
also works. These three alternatives are equivalent to DSSR internally.

As mentioned in the blog post Integrating DSSR into Jmol and PyMOL,
“The small size, zero configuration, extensive features, and robust performance make DSSR ideal to be integrated into other bioinformatics tools.” In addition to the DSSR-Jmol and DSSR-PyMOL integrations which I initiated and got personally involved, other bioinformatics resources are increasingly taking advantage of what DSSR has to offer. Here are a few examples:
Before aligning structures, STAR3D preprocesses PDB files with base-pairing annotation using either MC-Annotate (Gendron et al., 2001; Lemieux and Major, 2002) (for PDB inputs) or DSSR (Lu et al., 2015) (for PDB and mmCIF inputs) and pseudo-knot removal using RemovePseudoknots (Smit et al., 2008).
2014, RNApdbee: In order to facilitate a more comprehensive study, the webserver integrates the functionality of RNAView, MC-Annotate and 3DNA/DSSR, being the most common tools used for automated identification and classification of RNA base pairs.
2018, RNApdbee 2.0: Base pairs can be identified by 3DNA/DSSR (default) (4), RNAView (5), MC-Annotate (3) or newly added FR3D (15).
- The Universe of RNA Structures (URS) web-interface to the URS database (URSDB) makes extensive use of DSSR. For each analyzed structure (including PDB entries), the DSSR text output file (termed “DSSR-file”) is also available. Impressively, the maintainers of URS are quick with DSSR updates. The current version used by the URS website is DSSR v1.7.4-2018jan30.
Forty years after the yeast phenylalanine tRNA structure was solved, modified nucleotides should no longer be an issue for RNA structural analysis, especially for this classic molecule. Automatic processing of modified nucleotides is just one aspect of DSSR’s substantial set of features. Based on my understanding of the field, more structural bioinformatics resources/tools could benefit from DSSR. Simply put, if one’s project is related to 3D DNA or RNA structures, DSSR may be of certain help. It’s just a timing issue that DSSR would benefit a (much) larger community.

When visiting the RCSB PDB website today, I am please to notice that the PDB now contains “10015 Nucleic Acid Containing Structures”. Based on “Macromolecule Type” in “Advanced Search” of the RCSB PDB website, I observed the following information:
- The number of DNA-containing structures is
6,384
(reported in 2,997
papers), and the corresponding number for RNA-containing structures is 3,861
(associated with 2,012
publications).
- There are
4,570
structures containing both DNA and protein (potentially forming DNA-protein complexes), and 2,478
RNA-protein complexes.
- The smallest nucleic-acid-containg structures have only two nucleotides (e.g., 3rec), and largest ones are the ribosomes (and virus particles).
- The earliest released DNA structure from the PDB is 1zna (on March 18, 1981), a Z-DNA tetramer. The earliest RNA structure released is 4tna (on April 12, 1978), a refined structure of the yeast phenylalanine transfer RNA.
This landmark achievement is made possible by the world-wide scientific community through decades of efforts solving DNA/RNA 3D structures via experimental approaches (mainly solution NMR, x-ray crystal, and cryo-EM). These over 10K nucleic acid structures present both challenges and opportunities for the field of structural bioinformatics, especially for intricate RNA molecules. DSSR is an integrated software tool for dissecting the spatial structure of RNA. It is my effort in addressing the challenging issues for the analysis/annotation and visualization of RNA structures.

It is textbook knowledge that the Watson-Crick (WC) pairs are specific, forming only between A and T/U (A–T/U or T/U–A) or G and C (G–C or C–G). Furthermore, an A only forms one WC pair with a T, so is G vs. C. The widely used dot-bracket-notation (DBN) of DNA/RNA secondary structure depends crucially on this feature of specificity and uniqueness, by using matched parentheses to represent WC pairs, such as ((....))
for a GCGA (GNRA-type) tetra-loop of sequence GCGCGAGC
.
The reality is more complicated, even for what’s presumably to be a ‘simple’ question of deriving RNA secondary structure from 3D coordinates in PDB. One subtlety is related to the ambiguity of atomic coordinates that renders one base apparently forming two WC pairs with two other complementary bases. As always, the case can be best illustrated with a concrete example. The image shown below is taken from PDB entry 1qp5 where C20 (on chain B) forms two WC pairs, each with G4 and G5 (on chain A) respectively.
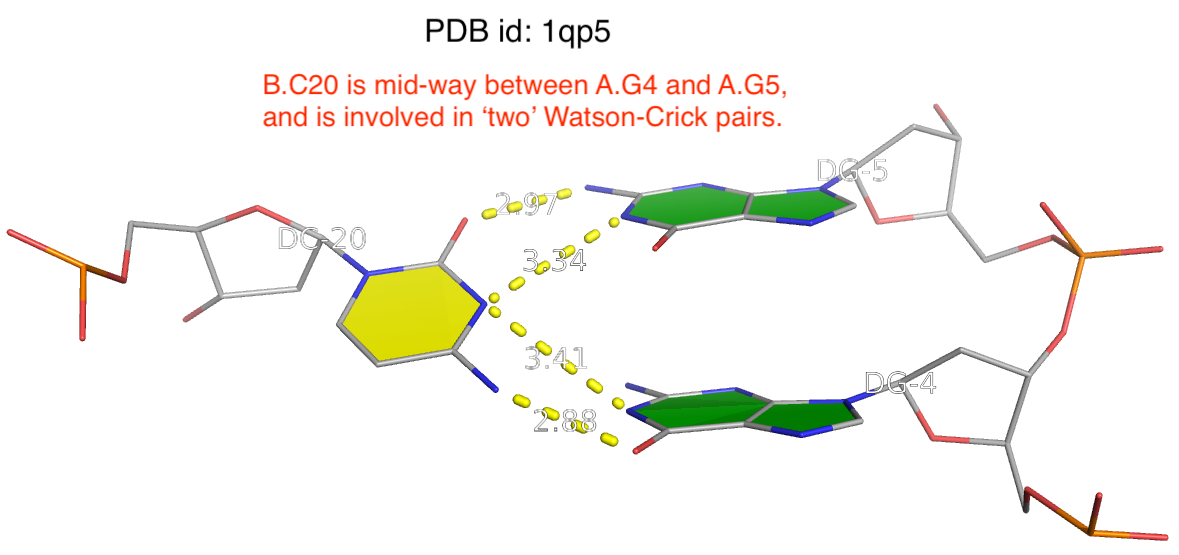
Clearly, taking both as valid WC G–C pairs would make the resultant DBN illegitimate. DSSR resolves such discrepancies by taking structural context into consideration to ensure that one base can only have a WC pair with another base. Here the G5–C20 WC pair is retained whilst the G4–C20 WC is removed.
This issue, one base can form two WC pairs as derived from the PDB, has been noticed for a long while. Two examples from literature are shown below:
The crystal structure data files were downloaded from the Research Collaboratory for Structural Bioinformatics (RCSB) Protein Data Bank (Berman et al. 2000). For each crystal structure, the set of canonical base pairs was extracted by selecting all Watson–Crick and standard G-U wobble pairs found by RNAview (Yang et al. 2003). Occasional conflicts in this list, where RNAview annotates two bases, x and y, as a standard base pair and also y and z as another conflicting base pair, were removed manually by visual inspection of the crystal structure in the program PyMOL (http://pymol.sourceforge.net/). The helix-extension data set was created by taking the canonical pairs and adding all additional base–base interactions identified by RNAview (excluding stacked bases and tertiary interactions) for which the direct neighbor was already in the collection. This means each base pair (i,j) was added if both i and j were still unpaired and if either (i + 1, j – 1) or (i –1, j + 1) were already in the set.
… From these complexes, we retrieved all RNA chains also marked as non-redundant by RNA3DHub. Each chain was annotated by FR3D. Because FR3D cannot analyze modified nucleotides or those with missing atoms, our present method does not include them either. If several models exist for a same chain, the first one only was considered. For the rest of this paper, the base pairs extracted from the FR3D annotations are those defined in the Leontis–Westhof geometric classification (24).
For each chain a secondary structure without pseudoknots was deduced from the annotated interactions, as follows. First all canonical Watson–Crick and wobble base pairs (i.e. A-U, G-C and G-U) were identified. Then, since many structures are naturally pseudoknotted, we used the K2N (25) implementation in the PyCogent (26) Python module to remove pseudoknots. Problems arise when a nucleotide is involved in several Watson–Crick base pairs (which is geometrically not feasible), probably due to an error of the automatic annotation. Those discrepancies were removed with a ad hoc algorithm such that if a nucleotide is involved in several Watson–Crick base pairs, we remove the base pair which belongs to the shortest helix.
By design, DSSR takes care of these ‘little details’, among other handy features (such as handling modified nucleotides and removing pseudoknots). By providing a robust infrastructure and comprehensive framework, DSSR allows users to focus on their research topics. If you have experience with other tools, such as RNAView and FR3D cited above, give DSSR a try: it may fit your needs better.
